The concept of a T-shaped professional is a good one. It suggests the need for both a breadth (top of the letter “T”) and depth (central pillar of the “T”) of skills to excel in your role.
McKinsey & Company adds “For any given role, some skill requirements are universal. Every team member may need to be comfortable working with data, or solving problems in a structured way, for example. Beyond those basics, however, they will also want to develop a deeper understanding of topics that allow them to make a real difference in their job… The result is a T-shaped skills profile, with a broad set of generally applicable skills, supplemented by a spike of specific expertise.”
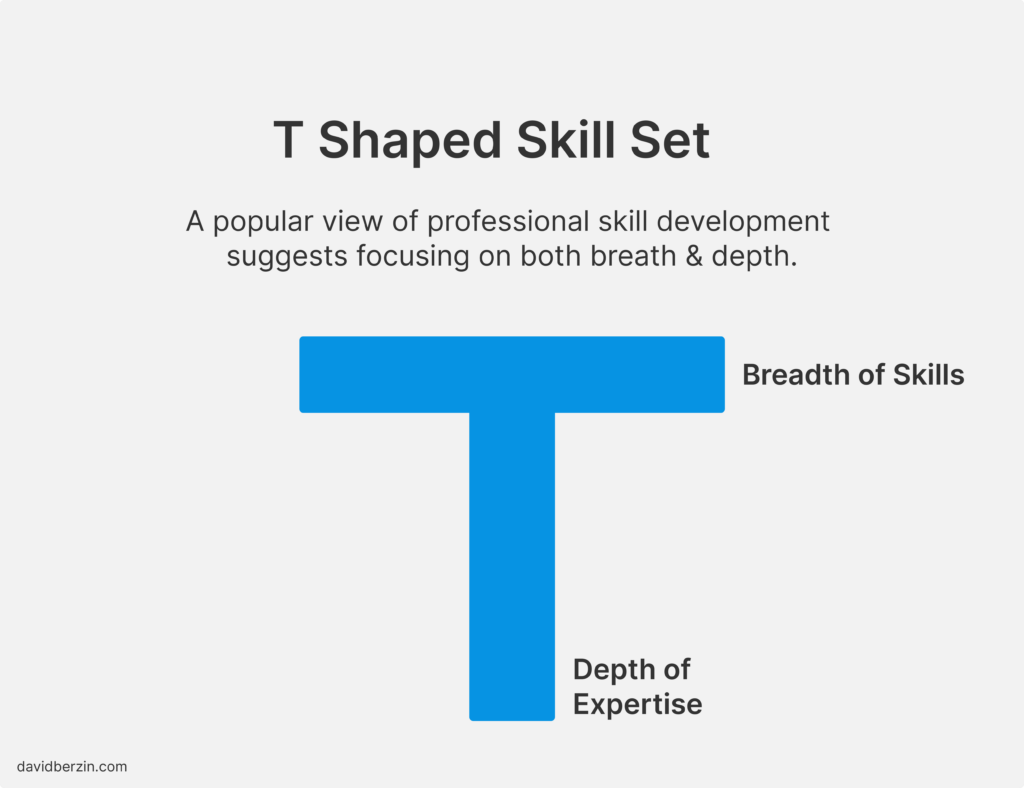
T-shaped skills are especially important in Product Management, a renaissance role that requires a mix of hard research/design/data/technical skills and softer collaboration/communication/consensus-building skills across diverse internal and external partners. In my product leadership roles, I work to develop both my own T-shaped skills and also to develop an organization of strong, diverse Ts that build something unique when combined together – the box-shaped product team – a synthesis of individual competencies that is more than the sum of its parts.
The Top of the T: The Default Product Manager Skill Set Curriculum
I love working in product management as it allows me to consistently leverage a highly diverse set of skills ranging from business strategy to experience design to quantitative analysis. There are parallels in my personal life. I’m a lifelong musician who started on the piano but then added guitar, bass, drums, production, vocals, and more.
Even better, the diverse skills of the PM are constantly changing. A decade ago, the archetype product manager was an ex-Google engineer, immersed in coding and architecture. Half a decade later, the pendulum swung to customer-centric experience design. Things have shifted again and now I consider the product role fundamentally a data/business one, albeit one that requires a constant conversation with operational, technical, design, quantitative, and other specialized teammates.
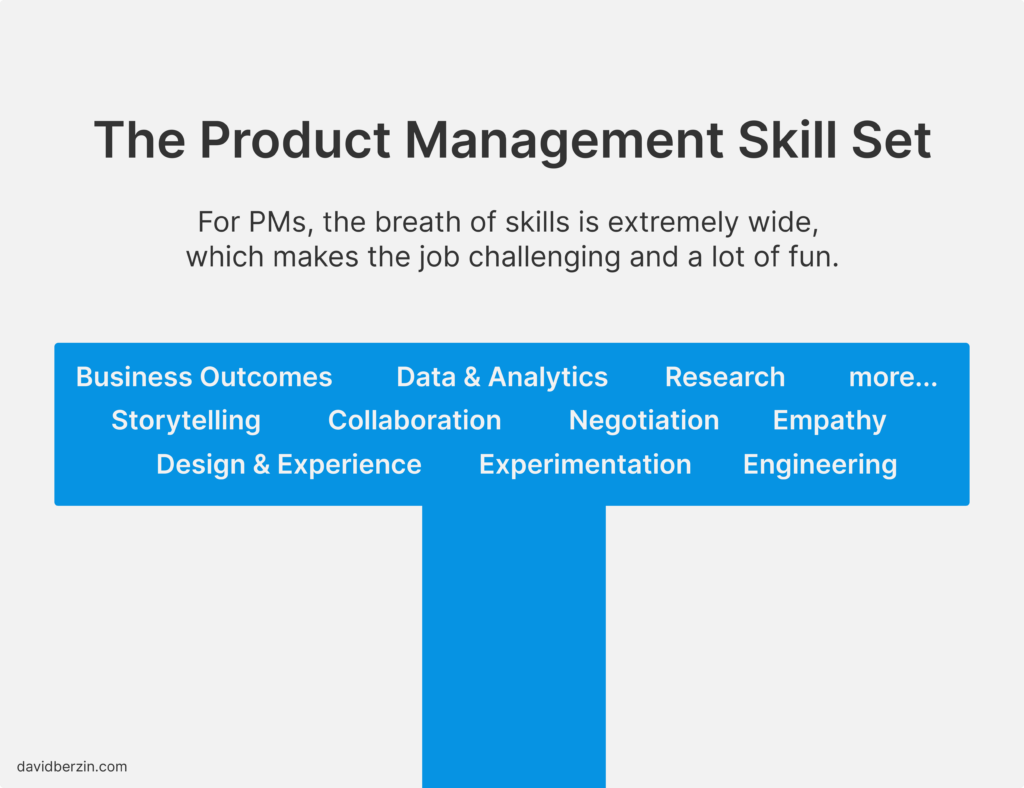
I actively engage my teams in an ongoing dialogue to define and refine the contents/curriculum of this “top T”, and push to foster a culture of continuous learning and development of these different skills through various means including 1:1s, pairing, book clubs, external mentorships, online education, and more.
The Pillar of the T: Each Team Member’s Specialization
Beyond the foundational skills, I encourage every team member to cultivate deep expertise in specific skill segments of the Product Management curriculum that resonate with their interests and abilities.
Teams are better when we fully support – and enjoy – each team member’s natural strengths and talent. This is why they’re here. Peter Drucker perhaps went overboard here when he suggested “Focusing on strengths is development, whereas focusing on weakness is damage control” and “to focus on one’s weakness at work is misuse, if not abuse of the person”, but Drucker certainly knew where the upside was.
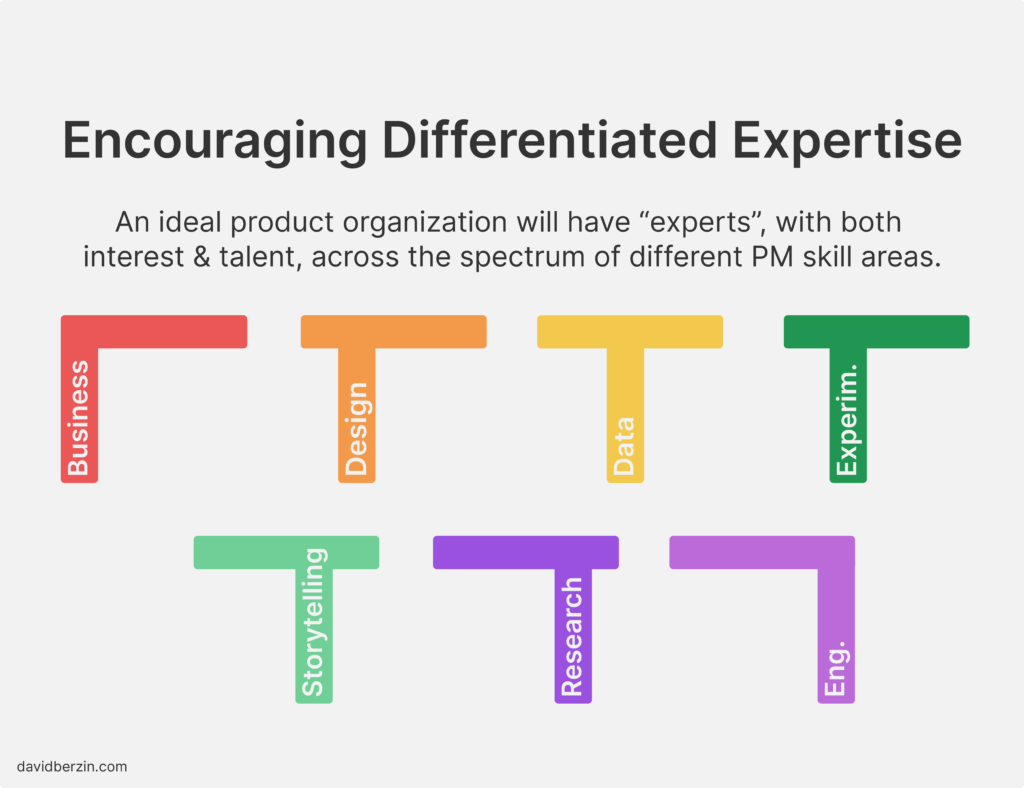
A case in point is my experience leading Viacom’s Social Data Product team. Each team member was encouraged to hone their unique strengths. Brian, with a technical predisposition, delved deep into algorithms alongside our data scientists. In contrast, Tanmay excelled in public speaking and presentation and refined those skills to perfection. The collective growth in individual specializations (plus foundational PM skills) up-leveled the overall impact of our team and both individuals to executive management roles.
A Super Team: A Symphony of Diverse Ts
The result of growing both the breadth and diverse depths of product management skills is a product team of specialized experts, with a common framework of foundational skills to get jobs done. The organization has superpowers all across the top of the T and teammates who are pursuing their passions, interests, and strengths. It’s a dynamic where every team member’s depth amplifies our collective capability, fostering an environment of shared learning and mutual growth.
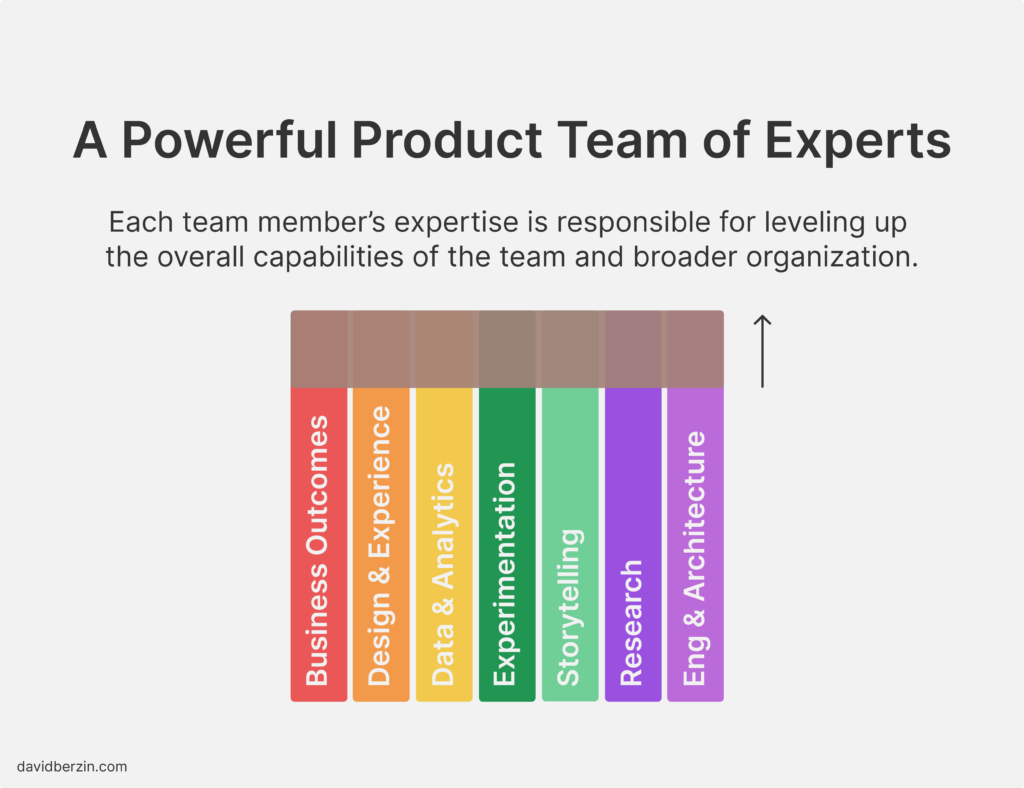
Together we form a box shape that falls outside of the English alphabet, with a rising, rock-solid top-of-the-T with deep strength across multiple functional areas.
Conclusion
In essence, the orchestration of T-shaped professionals into a cohesive unit is akin to a musical group of varied instruments creating a harmonious song. Each instrument, with its unique tone and timbre yet familiar with the score, contributes to the grandeur of the ensemble. Similarly, in the world of Product Management, the mix of shared skills and diverse specializations culminates in an organization that is skilled, innovative, and ready to tackle the multifaceted challenges ahead.