This is the second in a series of posts designed to help managers think about business requirements for selecting enterprise vendors and software. Please also check out my first post on Business Intelligence platforms.
Customer Data Platforms (CDPs) inspire a lot of confusion. Best to begin with what they are and what they are not.
CDPs are:
- A centralized platform for storing all of the user data about all of your users
- A platform that can be used by non technical employees to activate / action upon user data
- An safe-haven for secure user data management, compliant with the latest regulations and best practices
- A bridge to combine your user data with external data sets
- A rules engine for user segment management. Want to build cohorts of users who opened an email and clicked on a Facebook ad – no problem
- A platform for collaboration, breaking down individual business unit data silos
CDPs are not:
- CRM solutions designed for sales or support teams to manage intricate customer interactions and workflows
- DMP solutions focused only on anonymous cookied / IDed users (though they are coming close to covering this feature set)
- Tag Management solutions designed to wire up various vendor libraries and SDKs. Many CDPs were Tag Managers, but I think the historic focus on tag management is a disadvantage to be a best of breed CDP. Just because you were a horse, it doesn’t make you a better car
And why do people integrate Customer Data Platforms? Centralizing user data, strengthening the intelligence around it, and democratizing access to use it should impact business goals across the board from decreases systems costs to improved conversion rates.
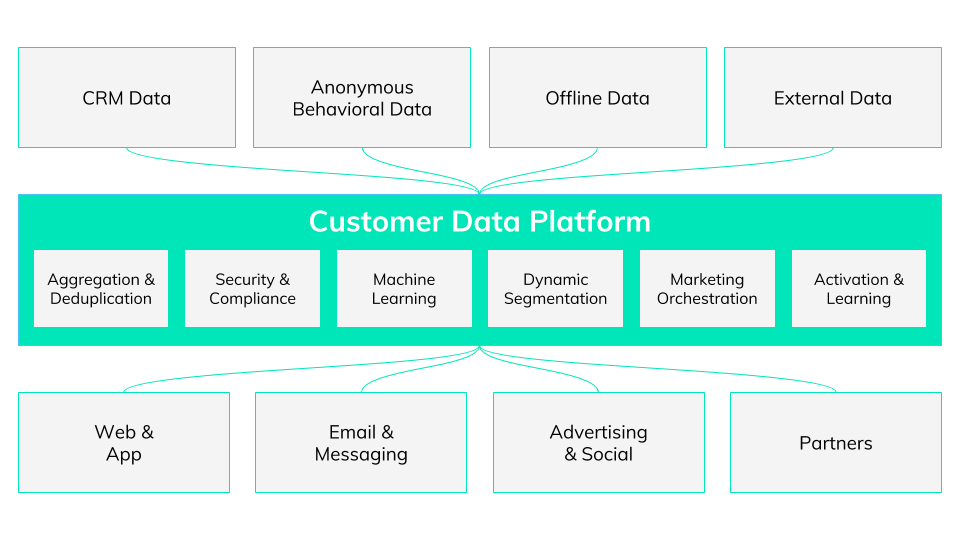
Given all of this, let’s review my Goldilocks (“just right”) criteria for picking a Customer Data Platform:
Connectivity and I/O
Customer Data Platforms are only as good as the pipes that bring data in and out of them. You want many different roads into the platform from plug and play SDKs / libraries to full read / write APIs. You also want pre built connectors into the most popular data sources (CRM, event ticketing platforms, etc) and data activation endpoints (ad networks, social media channels, email service providers, etc).
Security and Compliance
As we’ve learned over and over recently, user data security and governance is no easy tasks. Outsourcing this to a vendor may be a hard decision to make, but it’s often much harder managing and maintaining secure and compliant user data solutions internally. You want a partner with a tract record of secure data management, comparable customers that you trust and no fear of security audits from your team or others. You also want a partner that is quick to update to changing industry rules and regulations (ex. GDPR). Internally, you want robust rules, roles and permission settings to partition off sensitive data for specific users and use cases.
Administrative Usability
CDPs are designed to democratize data-driven activities for non-technical users. As such, you should require a modern, usable UX for non-engineers to get busy with the data. Some providers require light scripting for segment creation or segment activation. No good. Best to trail the administrative user experience with some of your least technical colleagues before pulling the trigger on a vendor solution.
Identity Management and Identity Resolution
There are a number of features in this functionality bucket, but in short, you want your CDP to consolidate literally all of your available user data into a singular user profile. This might mean partnering with a device or identity-graph provider to stitch emails to cookies.
This also means flexible data storage limits so that you don’t have to discard potentially valuable user data limits. At Viacom, a certain % of the US population visits our sites / websites or volunteers their email addresses. That said, our TV signals reach the homes and mobile devices of a much larger user base. We need systems to allow us to pull all of our data together without worry about a vendor’s storage costs or historic architectural limits.
Real Time Segmentation Updates
You user’s profiles and segments should update in real time as they take actions on and offline. Many CDPs update segments hourly – which is no bueno. If a user views / interacts with your website or an online ad, their profile should update immediately so they can activate to the next event in your funnel. Many of the CDPs who came from legacy industries (again, Tag Management) are just not architectured to support real time updates. This is of growing importance.
Integrated and Automated Machine Learning
The next generation CDPs go further than data storage and segment storage. The best support unstructured data and use machine learning to automatically create useful user segments. Some even crawl and categorize your content (pages, emails, posts) to find interesting patterns and apply those as dynamic segments to your users. This is the type of thinking you want to see from your Customer Data Platform partners.
The platform should also support custom data science models – whether run internally within the CDP or through easy and performant read / write APIs.
ML fanboy alert – this is one of my very top considerations when reviewing partners.
Smart Orchestration
Getting your users through a funnel from start to conversion is never easy. Your CDP should monitor and track your progress and where possible add dynamic intelligence to usher users through funnel events and towards your target goal. The alternative is intricate manual workflow creation and management, which is hard to set up and even harder to manage against other initiatives.
This dynamic orchestration allows for truly personalized, omni-channel user journeys – experiences and messages that change based on the individual user’s profile properties and the best likelihood of conversion.
Industry Momentum
There is a ton of investment in the CDP space right now. You’ll want to pick a horse with recent major funding from venture capital or a strategic investors. Many of these companies will not be in business in two year’s time.
-David