I stopped by the new Apple store in Williamsburg Brooklyn today in search of answers. First up was the quest for new large format display to plug my laptop in to when I’m fixed at home and need the extra space. “We no longer sell them. Here’s an LG display; this one’s great cause it has built in speakers”. Then I checked out the new 15″ laptops, as large format wins since working ergonomics beat carrying ergonomics (?), “This one has an y processor, but ram is capped at y and costs x”, “This one costs 2x and is the right call”. I asked why pick the more expensive one, and I believe the answers included better trackpad, speaker positions and a non-mobile processor. Meanwhile, my latest iPhone crapped out and required a reboot.
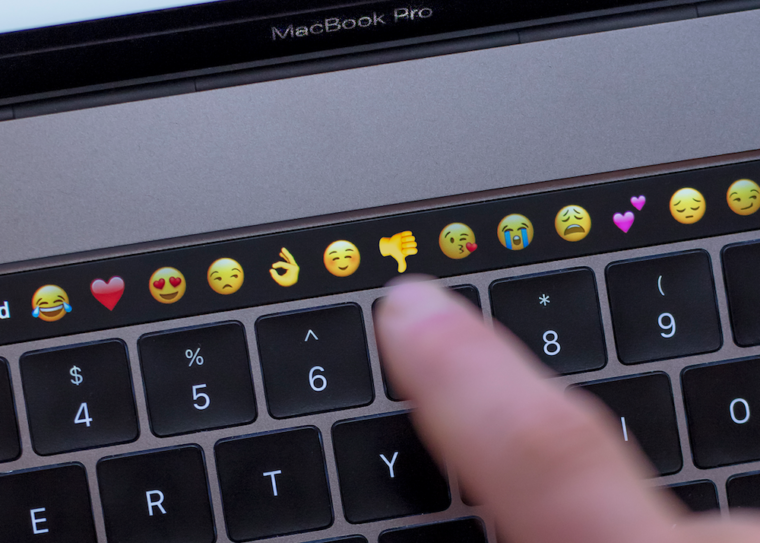
About Steve Jobs. This guy, from the little I’ve read – which does not include any official or unofficial biographies – was a maniac. Criticism should start with his disregard for the human condition of his workers, then perhaps move to the heavy metals used in his manufacturing (yes, Apple’s push towards recyclability and other efforts were better than most). But let’s focus on his management style – brutal, dominating, Amazon-esque and such.
All disclaimed, a question surfaces — was this a golden age for product? Shit just worked and it was elegant as fuck. Of course, my new phone takes photos with a gazillion more megapixels, but it does crash more than the dickens. I can’t open my current iPhone without “Voice Assist” (I see you Siri) offering it’s mind-numbing help. I can’t dock my laptop into my display without creating massive confusion during the next video conference. The list goes on, but it all circles back to Steve.
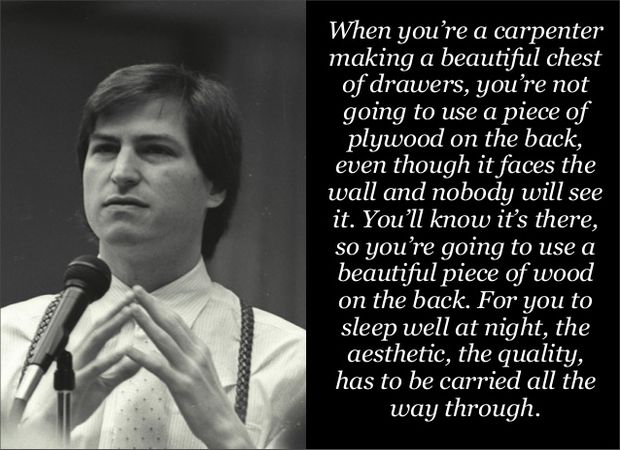
Does a product owner need to be maniacal to be effective? Did Steve need to be a dick to be great? Did jr. developers and sr. accounts needs to be rolled under his tires for my iPhone to simply work properly when I asked it to?
My oldest friend worked in the kitchens of some of the world’s best restaurants, and commented that the culture there was abusive and awful. I do not condone any of that, honestly, but I do recognize the context of ‘worlds best’. Do 20 unhappy campers – who get to list Noma or Apple on their resume – undo the experience of thousands or hundred of millions of impressed customers? Whats the balance?
Hard to say. I’m now open to shop for non-Apple products, but I’m fairly sure the options are meh.